The Importance of Machine Learning Annotation for Businesses
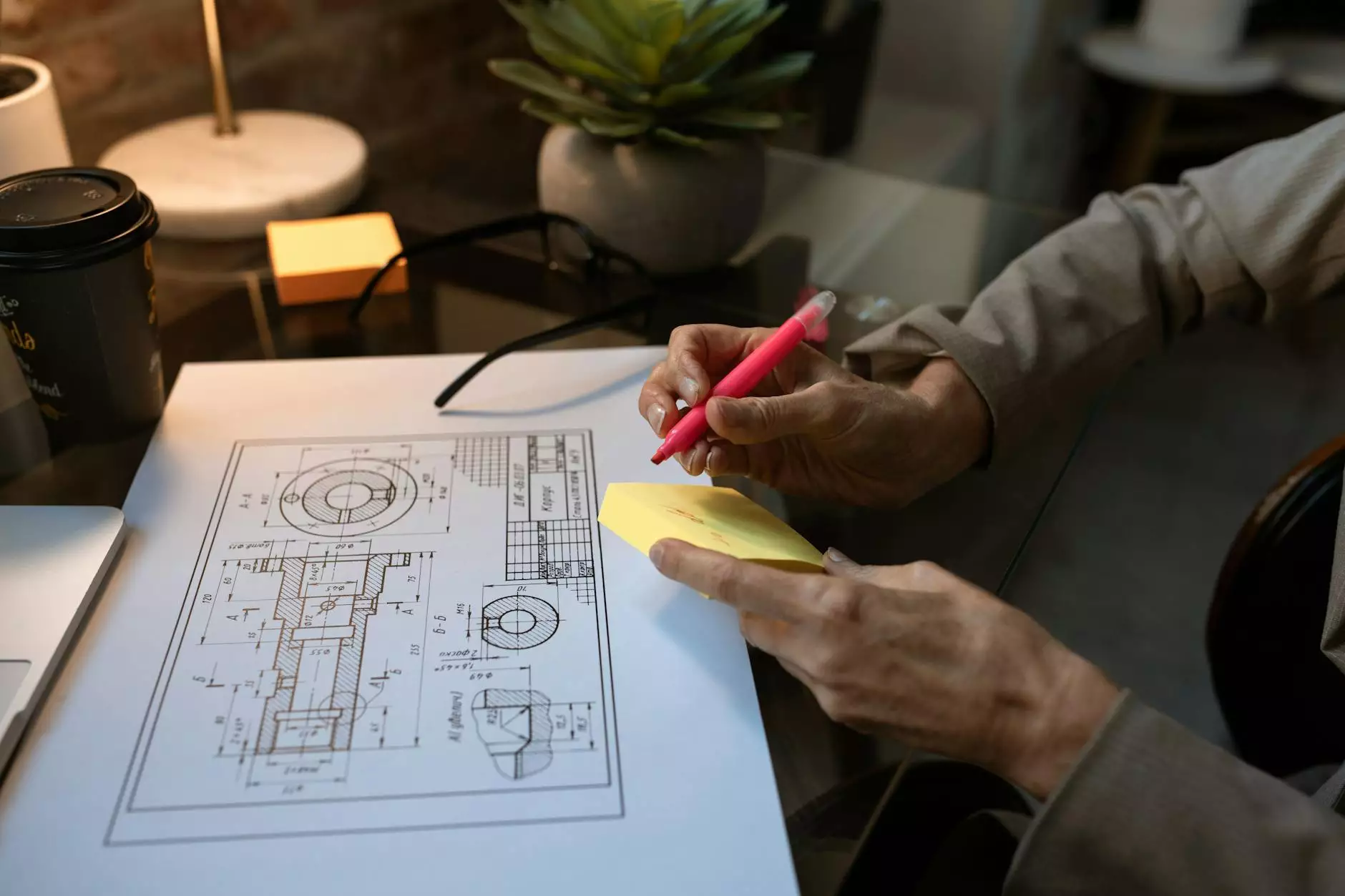
In today's fast-paced digital landscape, businesses are continually seeking ways to enhance their operations and improve customer experiences. One significant technology revolutionizing various industries is machine learning. Central to the success of machine learning applications is the process known as machine learning annotation. This article explores the vital role of annotation in unlocking the full potential of machine learning, particularly for businesses in the Home Services and Keys & Locksmiths sectors.
Understanding Machine Learning
Machine learning combines data with algorithms to enable systems to learn and improve from experience without being explicitly programmed. It allows businesses to make predictions, identify patterns, and automate decision-making processes. However, to function optimally, machine learning models require high-quality, annotated data.
What is Machine Learning Annotation?
Machine learning annotation is the practice of labeling and organizing data so that machine learning algorithms can understand and learn from it. This process is critical as the quality and accuracy of the annotations directly affect the performance of the machine learning models. Properly annotated data helps in training algorithms to recognize features and predict outcomes effectively.
Types of Data Used in Annotation
Machine learning models can learn from various types of data. Some common types include:
- Text Data: Annotations help in sentiment analysis, spam detection, and customer feedback analysis.
- Image Data: Annotating images allows models to identify objects, detect anomalies, and improve security surveillance systems.
- Audio Data: Annotations are necessary for voice recognition, transcription, and musical style classification.
- Video Data: Used for activity recognition, event detection, and security monitoring.
Why is Machine Learning Annotation Crucial for Your Business?
Businesses, especially those in the Home Services and Keys & Locksmiths sectors, can reap substantial benefits from effective machine learning annotation. Below are several reasons highlighting its significance:
1. Enhanced Decision Making
Annotated data enhances the capability of machine learning algorithms to analyze past trends and predict future outcomes. For instance, locksmiths can predict peak business hours based on historical request data, allowing for better staffing and resource allocation.
2. Improved Customer Experience
In industries like home services, timely and accurate responses to customer inquiries are crucial. Machine learning can be trained on annotated customer interactions to provide automated responses or recommendations, ensuring that customers receive prompt service.
3. Increased Operational Efficiency
Annotating data can help identify inefficiencies in service delivery. For example, by analyzing service response times through annotated data, businesses can streamline their processes, reducing wait times and improving overall efficiency.
4. Personalized Marketing Strategies
With annotated data, businesses can segment their audience more effectively and tailor marketing strategies based on customer preferences and behaviors. This targeted approach can lead to higher conversion rates and improved customer loyalty.
How to Implement Machine Learning Annotation in Your Business
Integrating machine learning annotation into your business processes may seem daunting, but it can be streamlined through the following steps:
Step 1: Identify Data Needs
Determine the types of data relevant to your business. For a locksmith, this might include customer inquiries, service records, and equipment usage logs. Understanding your data needs is the foundation for effective annotation.
Step 2: Choose the Right Tools and Technologies
Select appropriate tools for annotation, such as desktop applications, web-based platforms, or even automated annotation tools that leverage AI. Depending on your budget and expertise, you may consider various options.
Step 3: Utilize Human and Machine Resources
A hybrid approach often yields the best results. While automated tools can speed up the process, human annotators ensure accuracy and high-quality outputs. Businesses can employ skilled professionals to annotate critical data points while using tools for larger datasets.
Step 4: Train Your Machine Learning Models
Once the data is annotated, it is time to feed it into machine learning models for training. This training process helps machines learn from the data, improving their predictive capabilities. Regularly retraining models with new annotations is crucial for maintaining accuracy.
Step 5: Monitor and Optimize
After deployment, continually monitor the performance of your machine learning models. Collect feedback and adapt the annotation process as necessary. Business needs evolve over time, and staying responsive to changes will help maintain the model's relevance and accuracy.
Challenges in Machine Learning Annotation
Despite its significant advantages, machine learning annotation presents certain challenges:
- Data Privacy Concerns: Sensitive customer data must be handled carefully to ensure compliance with privacy regulations.
- Consistency and Accuracy: Variability in how different annotators label data can lead to inconsistencies, adversely affecting model performance.
- Scalability: As businesses grow, the volume of data requiring annotation can become overwhelming, necessitating scalable solutions.
The Future of Machine Learning Annotation in Business
The future of machine learning annotation is bright, with ongoing advancements that promise to enhance how businesses leverage machine learning. Emerging technologies such as crowdsourcing annotation, automated tools employing AI, and improved machine learning algorithms are set to reduce the burden of annotation while improving accuracy.
Innovations on the Horizon
Several exciting innovations in the annotation field are likely to impact businesses significantly:
- Crowdsourcing: This model allows businesses to tap into a global workforce for data annotation, reducing costs and increasing throughput.
- Active Learning: Machines can identify uncertain data points and request human annotation only when necessary, optimizing resources.
- Real-Time Annotation: With advancements in AI, real-time data annotation for applications like live surveillance or customer service has become increasingly feasible.
Conclusion
In conclusion, machine learning annotation is a critical component for businesses seeking to leverage the power of machine learning effectively. For Home Services and Keys & Locksmiths industries, annotated data can lead to enhanced decision-making, improved customer experiences, and increased operational efficiency. By understanding the fundamentals of data annotation and its application in machine learning, businesses can position themselves for long-term success in an increasingly automated and data-driven world.
Invest in quality machine learning annotation today and watch your business reap the benefits of more informed decision-making and a deeper understanding of your customer base.