Unlocking Business Potential: The Role of Machine Learning Data Labeling
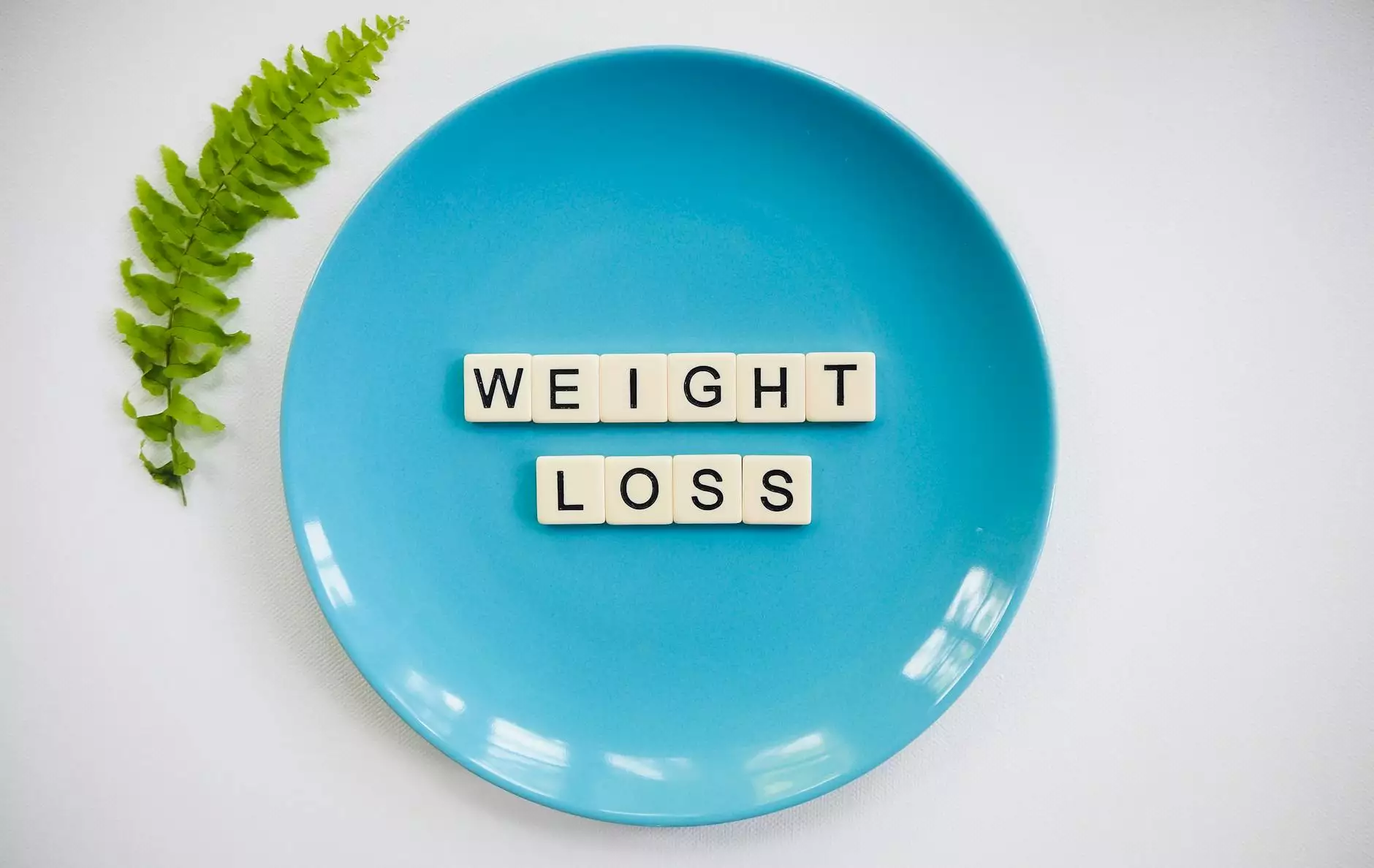
In an era where machine learning has become a driving force behind technological advancements, businesses such as those in the Home Services and Keys & Locksmiths sectors must harness this power to stay competitive. One of the critical components of machine learning applications is data labeling. This article delves into the significance of machine learning data labeling, its impact on business efficiency, and how it can be leveraged to optimize services effectively.
Understanding Data Labeling
Data labeling refers to the process of annotating training data to help machine learning models learn from that data. For instance, a dataset of images might need labels indicating whether each image contains a locksmith's tools, makes of keys, or types of locks. In business, particularly in the fields related to home services and security, accurate data labeling is essential for machine learning algorithms which identify trends, predict requirements, and automate processes.
The Importance of Machine Learning in Business
Machine learning has revolutionized how businesses operate. By leveraging algorithms that learn from data, organizations can make more informed decisions, enhance customer experiences, and develop tailored solutions. Key benefits include:
- Cost Reduction: Automation of processes reduces the need for extensive manual labor.
- Improved Efficiency: Machine learning models can analyze large datasets faster than humans.
- Enhanced Customer Insights: Understanding customer needs through analyzed data leads to better service provisions.
- Predictive Maintenance: In the locksmith industry, predicting when a lock may fail and preparing in advance can save time and increase customer satisfaction.
Key Applications of Machine Learning Data Labeling in Home Services and Locksmiths
Businesses in the home services sector can utilize machine learning data labeling in the following ways:
1. Service Personalization
Data labeling enables businesses to create customer profiles based on preferences and past interactions. By analyzing labeled data, locksmiths can offer tailored solutions, such as recommending specific lock types based on a customer’s home security needs.
2. Automated Customer Support
Using labeled datasets, businesses can train chatbots to respond effectively to customer inquiries. For instance, a home services provider can automate responses for common questions related to service availability or pricing.
3. Improved Marketing Strategies
With correctly labeled data, companies can identify their target demographics and create effective marketing strategies. This can include targeting customers who are likely to need locksmith services based on geographical and behavioral data.
4. Risk Assessment and Fraud Detection
In the locksmith industry, machine learning can analyze transaction data for irregular patterns. By labeling data as 'normal' or 'suspicious', businesses can flag potential fraud and take precautions proactively.
Choosing the Right Data Labeling Strategy
Implementing machine learning data labeling requires a well-thought-out strategy. Here are some factors to consider:
- Quality of Data: Ensure your data is comprehensive and relevant to your business needs.
- Source of Data: Determine whether your data will be sourced internally or externally, and manage the labeling process accordingly.
- Labeling Tools: Invest in high-quality tools and platforms that streamline the labeling process.
- Expertise: Having skilled personnel for data labeling can significantly improve the accuracy and usability of the labeled data.
Best Practices for Data Labeling
To maximize the benefits of machine learning data labeling, follow these best practices:
1. Define Clear Guidelines
Establish clear instructions for how data should be labeled. This may include defining what constitutes types of locks, key styles, or specific customer scenarios.
2. Utilize Automation Where Possible
Consider leveraging automated tools for initial data labeling, followed by human verification to ensure accuracy.
3. Regular Quality Assurance
Conduct periodic reviews of labeled data to ensure consistency and accuracy. This is crucial for maintaining high-quality training datasets.
4. Continuous Training for Labelers
Regular training sessions for data labelers can help them keep up with trends and ensure they understand the evolving needs of the business.
Measuring Success: Analyzing Labeling Effectiveness
To determine the effectiveness of your data labeling efforts, establish metrics for success. Consider metrics such as:
- Labeling Accuracy: The percentage of correctly labeled data.
- Time Efficiency: The average time taken to label the data.
- Impact on Business Outcomes: Analyze how labeled data has positively affected service delivery, customer satisfaction, or sales.
Future Trends in Machine Learning Data Labeling
The future of machine learning data labeling is poised for significant advancements. Some of the trends include:
1. Increased Use of Synthetic Data
Synthetic data generated by simulation could reduce the reliance on actual data, thus easing the labeling burden.
2. Advancements in Natural Language Processing (NLP)
NLP technologies will enhance how locksmiths can interpret unstructured data, such as customer reviews or feedback, leading to smarter business decisions.
3. Crowdsourced Labeling
Leveraging crowdsourcing can help businesses scale their labeling efforts while ensuring diverse input for richer datasets.
4. Shift Towards Self-Service Machine Learning
As more user-friendly machine learning tools become available, businesses will be able to manage their data labeling processes more autonomously.
Conclusion: Embracing the Future of Data Labeling for Business Growth
Integrating machine learning data labeling into business operations is an opportunity for companies in the home services and locksmith industries to boost efficiency, enhance customer service, and stay ahead of the competition. By investing in high-quality data labeling practices, businesses can unlock valuable insights and drive sustainable growth. As technology evolves, staying informed and adaptable will be key to successful implementation. Thus, understanding and applying of machine learning data labeling is not just an option—it's a necessity for future-proofing business strategies.